personal infos
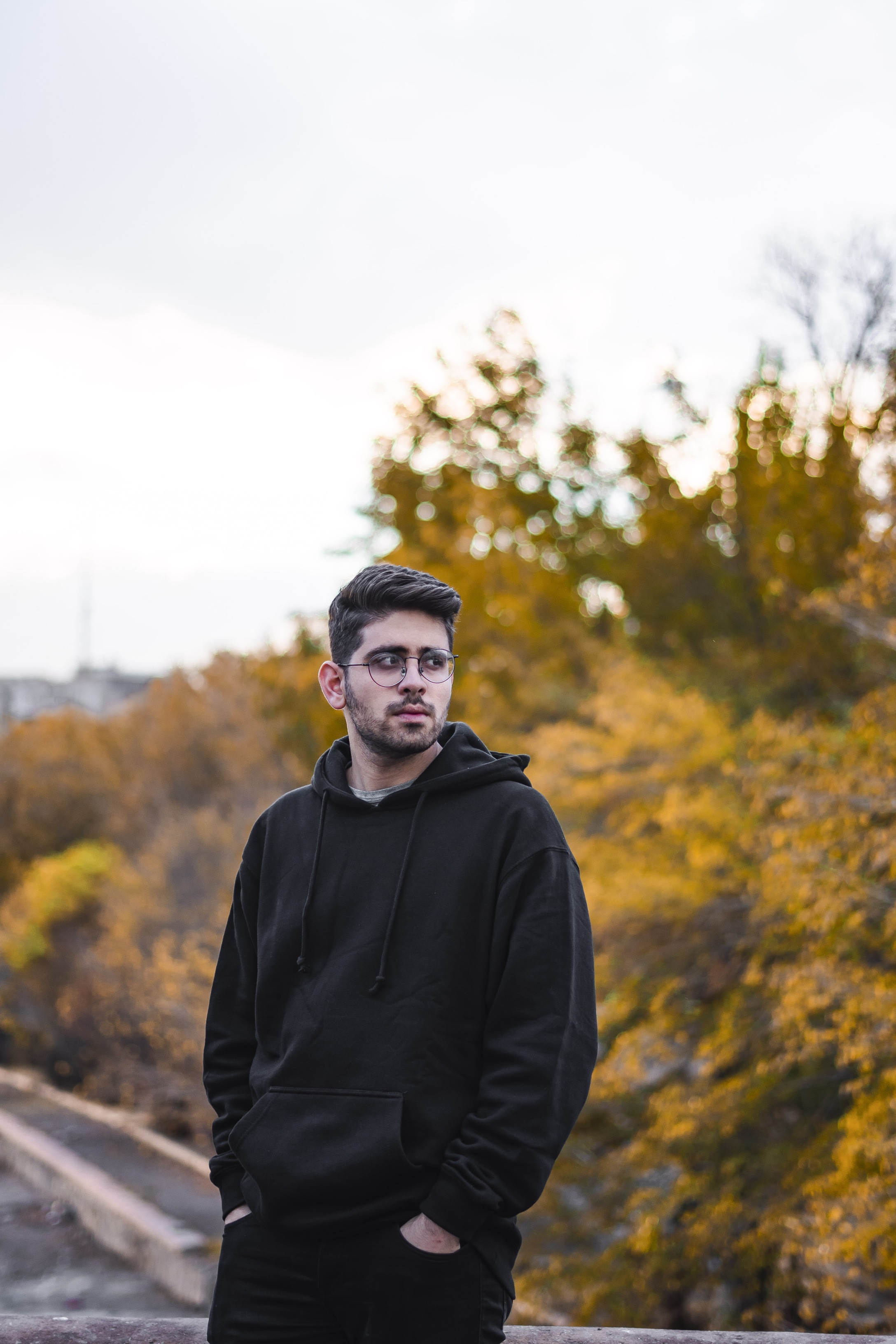
- First Name : Aydin
- Last Name : Ebrahimi
- Age : 27 Years
- Nationality : Iranian
- Freelance : Available for hire
- Address : Tabriz, Iran
- Phone : -
- Email : Aydinebrahimi1998@gmail.com
- Skype : Aydinebrahimi
- Languages : Turkish, English, Persian, Dutch
5
years of experience
14
completed projects
5
Publication
7
Certification
My Skills
Python
Google Earth Engine
Matlab
R Programming
ArcGIS
Global Mapper
SNAP
Cloud Compare
QGIS
Adobe Photoshop
Adobe Premiere Pro
Adobe Lightroom
Adobe After Effects
Publication & Education
-
Comparative Analysis of Deep Neural Networks and Traditional Machine Learning Methods for Land Cover Classification Using High Resoultion Remote Sensing Imagery Submitted to ISPRS 8th Geospatial Conference
Recent advancements in deep learning have led to significant improvements across various fields, including computer vision, biomedical engineering, and geospatial analysis. Deep neural networks (DNNs) are prioir at extracting complex spatial features from large-scale data, enabling more accurate automated interpretation of remote sensing imagery. Land cover classification has become increasingly critical due to applications in urban development, environmental monitoring, agricultural planning. As high-resolution satellite data become more accessible, demand for precise classification methods continues to grow. This study investigates DNN performance for land cover classification using high-resolution (50 cm) DigitalGlobe satellite imagery with three bands (R, G, B), pecifically using the DeepGlobe Land Cover Classification dataset derived from WorldView-3 satellite sensor imagery. We focus on six land cover classes: urban land, agricultural land, rangeland, forest land, water, and barren land. The dataset is divided into 70% training, 20% validation, and 10% testing subsets. Deep learning models (AttUnet, ResUnet++, and DeepLabV3+) are implemented and evaluated against traditional machine learning algorithms including Random Forest and Support Vector Machines. Evaluation metrics include overall accuracy, F1-score, and recall. We analyze test data using trained models and compare results to traditional algorithms to assess robustness and generalization capabilities under varying landscape complexities. We expect DNNs to outperform classic machine learning algorithms, significantly improving classification accuracy and spatial consistency, particularly in heterogeneous regions, though requiring greater computational resources and annotated data. This comparative analysis highlights practical considerations in selecting between conventional and modern classification approaches, contributing to ongoing efforts to advance intelligent, data-driven land cover mapping in remote sensing workflows.
-
Comparative Analysis for Post-earthquake Road Debris Detection Based on Deep Neural Networks Using High-resolution Remote Sensing Imagery Submitted to ISPRS 8th Geospatial Conference
Earthquakes can cause considerable damage to transportation infrastructure, affecting emergency response and recovery operations. Timely detection of road debris is important in such situations. The purpose of this research is to address the challenges related to the rapid detection of collapsed areas and road blockages, in order to support timely cleanup efforts and assist affected communities in responding to the damage. Deep learning has become an essential tool in remote sensing and image processing, offering improved capabilities for feature extraction, classification, and segmentation from complex and high-resolution imagery. Its integration into post-disaster analysis enables faster and more accurate assessments compared to traditional manual methods. This study examines the performance of three commonly used semantic segmentation models—Unet, Attention Unet (AttUnet), and ResUnet++—for road debris detection based on high-resolution satellite and drone imagery. Two earthquake events were used as case studies: the 2023 earthquake in Osmaniye, Türkiye (Mw 7.8), captured by the Pleiades satellite, and the 2017 earthquake in Sarpol-e Zahab, Kermanshah, Iran (Mw 7.3), captured by a Phantom 4 Pro drone. The models were evaluated using three metrics: Intersection over Union (IoU), Recall, and Accuracy. The results show that ResUnet++ achieved higher performance compared to Unet and AttUnet in both cases. For the Osmaniye dataset, ResUnet++ reached an IoU of 80.81%, Recall of 78.88%, and Accuracy of 96.12%. On the Sarpol-e Zahab dataset, it obtained an IoU of 81.62%, Recall of 80.28%, and Accuracy of 97.24%. Unet and AttUnet performed at lower levels across all evaluated metrics. This comparison provides a clear assessment of model performance in post-earthquake debris detection tasks and contributes to ongoing work in the application of deep learning and high-resolution imagery for geospatial analysis in disaster response contexts.
-
Post-earthquake road debris detection based on SEA-Unet using post-event Very High-resolution Remote Sensing Imagery: Case studies in Osmaniye, Türkiye and Sarpol-e Zahab, Iran. Submitted to Big Earth Data journal
Natural disasters, such as earthquakes, cause significant damage to the infrastructure and transportation systems of the affected areas. Rapid and accurate identification of road debris is imperative for practical post-disaster response efforts. Traditional methodologies involving field surveys and visual inspections are time-intensive and may hinder fast response. Leveraging recent developments in remote sensing (RS) technology and deep learning (DL), this study introduces a novel DL-based method, SEA-Unet, for automating road debris detection using Very High-Resolution (VHR) RS imagery. The SEA-Unet framework integrates the Squeezed and Excitation attention (SEA) mechanism and dense skip connections to improve accuracy and efficiency in debris detection. SE attention enables DL models to focus on critical features, substantially enhancing debris detection precision. We validate the proposed SEA-Unet methodology using VHR satellite imagery from the 2023 Osmaniye, Türkiye earthquake (magnitude 7.8, Pleiades acquisition on February 6, 2023) and the 2017 Sarpol-e Zahab, Kermanshah, Iran earthquake (magnitude 7.3 Mw, captured by Phantom 4 Pro). Comparative analysis demonstrated superior performance of SEA-Unet (mIoU=92.68%, Recall=88.39%, Accuracy=97.54% for Osmaniye, Türkiye, and mIoU=93.78%, Recall=89.23%, Accuracy=98.45% for Sarpol-e Zahab, Iran) over well- known models like Unet, ResUnet++, DeepLabV3+, Segnet, and ResUnet. This study shows the efficacy of SE attention in augmenting CNN models for high-precision image analysis, offering a significant advancement in post-disaster debris detection and response capabilities.
-
Improving the YOLOv5 Deep Neural Network for Detecting Vehicles and Outdoor Pools from Drone Data. Journal of Geomatics and Technology
Abstract : Detecting small objects such as vehicles and swimming pools in high-spatial-resolution drone images is challenging due to their similar geometric and color features. The increase in the number of vehicles is not only a major challenge from the perspective of urban traffic but also leads to environmental problems such as pollution and warming. Therefore, monitoring these targets can play an important role in managing these problems. On the other hand, the construction and maintenance of swimming pools also require a significant amount of water, and monitoring these targets in urban environments is essential for water conservation. In this regard, drone remote sensing images and deep learning networks, which have a high ability to detect objects from these images, are considered suitable tools for monitoring these targets. Although valuable research has been done in this area to address each of the environmental challenges mentioned, there are still shortcomings in them. In this study, a new deep learning network YOLOv5+ has been developed to simultaneously detect two targets, vehicles and swimming pools, from drone images, in which the network's performance in extracting efficient features has been enhanced due to the use of the Inception mechanism in the intermediate layers. Additionally, in this study, DJI Mavic and DJI Mini Se drone data from Tianjin regions in China and the city of Cannes in France were used to evaluate the performance of the proposed network and compare it with the YOLOv5 and YOLOv7 deep learning networks. Finally, the results showed that the proposed network achieved an overall accuracy of 95% on the test set, which is an improvement of 2% over the YOLOv5 and YOLOv7 networks, indicating the efficiency of the approach proposed in this study.
-
MASTER DEGREE Khaje Nasir Toosi University of Technology , Tehran, Iran
MSc. in Remote Sensing, GPA: 3.42/4 Master Thesis: Post-earthquake road debris detection based on deep neural networks and post-event high-resolution image
-
BACHELOR DEGREE University of Tabriz
BSc. in Geomatics, GPA: 3.4/4, Bachelor Thesis: Examining the displacement diagram of the planes of the surveyed points in 2 intervals at different times and breaking points.